Glossary
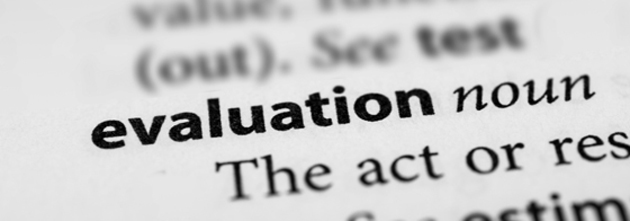
R
Randomised control study
Randomised control studies are a type of evaluation based on the experimental method. Since 1944, the year when the results of the British Medical Research Council's experiment on patulin were published, it has been a cornerstone of clinical research in the medical field. In clearly defined cases and with numerous limitations, randomized controlled trials (RCTs) can also be applied to the evaluation of public policies.
The method consists of dividing a group of individuals into two sub-groups by assigning members to the groups in a strictly random manner. The members of the first group, called the "experimental" group, receive the "treatment" (for example, a policy to deliver a service or grant a benefit). The members of the second group, the "control" group, are excluded from the treatment (the policy). If the initial sample is large enough, the two groups thus created are statistically equivalent in terms of both observable and non-observable characteristics. This means that the two sub-groups have no differences at the outset and that any selection bias is eliminated. The control group thus created is the most reliable reconstruction of the counterfactual situation.
This makes it possible to estimate the effect of the treatment simply by comparing the average value of the result variable in the two sub-groups.
The desirable statistical characteristics make this method an ideal benchmark in the counterfactual evaluation of public policies.
Regression analysis
Regression is an analysis tool based on the idea that observations relating to two variables can be represented on the Cartesian plane as a cloud of points to which lines or curves can be fitted. These interpret the trend and thus represent the relationship among the variables.
In linear regression, the line to which the points are fitted is determined by minimising the square of the distances between the points and all possible lines crossing the cloud (method of least squares).
When evaluating the effects of a policy, regression is used to reduce the initial differences when treated and non-treated samples are compared. However, it can also be used in the experimental method to eliminate residual differences between the control group and the experimental group and to increase the precision of the estimates.
If we include in the regression the variables that are deemed to influence both the allocation of the treatment and the variable chosen to measure its effect, we obtain a final estimate of the effect "under equal conditions". In general, therefore, the more variables influencing the selection process that can be included, the more plausible the estimates will be.
If the treatment variable is binary (treated-not treated) and the regression is based on pre- and post-treatment data, the estimates obtained correspond to those of the "difference in differences" (DID) model.